R for Data Science Exercises: Workflow Code Style and Data Tidying
These exercises are focussed on furthering your workflow coding style and tidying messy data to make analysis easier.
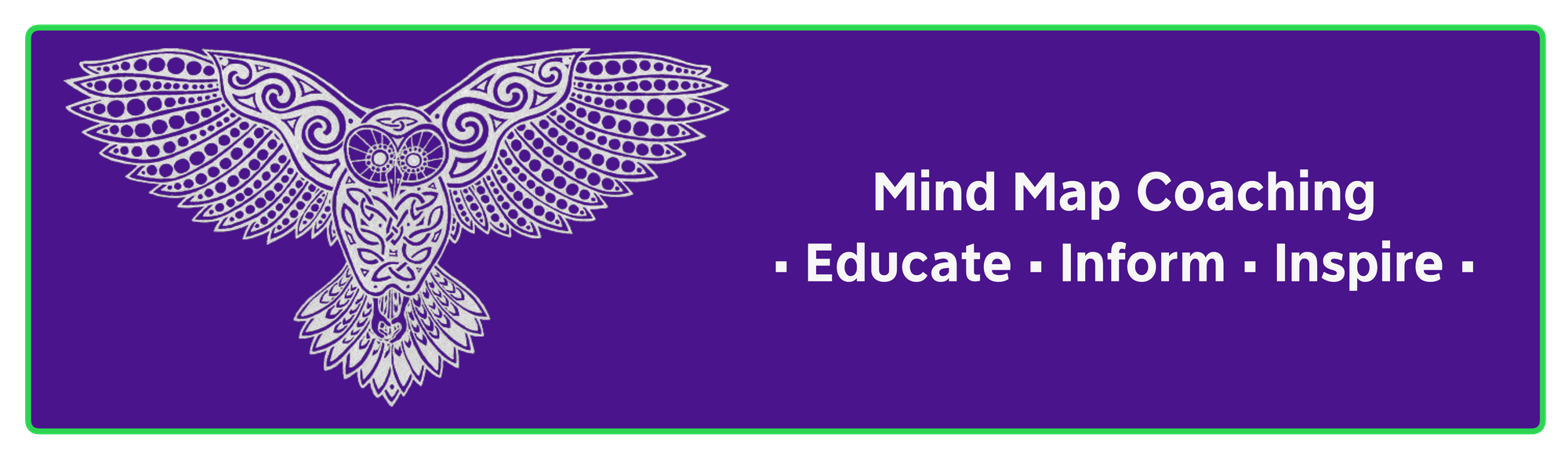
R for Data Science 2nd Edition Exercises (Wickham, Mine Çetinkaya-Rundel and Grolemund, 2023)
Workflow Code Style
Run the code in your script for the answers! I'm just exploring as I go.
Workflow Code Style Exercises
Packages to load
library(nycflights13)
library(tidyverse)
- Restyle the following pipelines using what you have learned in this section:
#1
flights|>filter(dest=="IAH")|>group_by(year,month,day)|>summarize(n=n(),
delay=mean(arr_delay,na.rm=TRUE))|>filter(n>10)
#2
flights|>filter(carrier=="UA",dest%in%c("IAH","HOU"),sched_dep_time>
0900,sched_arr_time<2000)|>group_by(flight)|>summarize(delay=mean(
arr_delay,na.rm=TRUE),cancelled=sum(is.na(arr_delay)),n=n())|>filter(n>10)
-
Restyling:
-
Insert a
space
before and after each pipe operator (|>
). -
Insert a
linebreak
after each pipe operator (|>
). -
Insert a
space
before and after all operators in the code==
,=
,>
,<
,,
.
-
#1
flights |>
filter(dest == "IAH") |>
group_by(year, month, day) |>
summarize(
n = n(),
delay = mean(arr_delay, na.rm = TRUE)
) |>
filter(n > 10)
#2
flights |>
filter(
carrier == "UA",
dest %in% c("IAH", "HOU"),
sched_dep_time > 0900,
sched_arr_time < 2000
) |>
group_by(flight) |>
summarize(
delay = mean(arr_delay, na.rm = TRUE),
cancelled = sum(is.na(arr_delay)), n = n()
) |>
filter(n > 10)
Data Tidying
Data Tidying Exercises
Packages to load
library(tidyverse)
Clarifications:
-
variables
= columns. -
observations
= rows. -
values
= cells.
tidyr
provides two functions for pivoting data: pivot_longer()
and pivot_wider()
.
-
pivot_longer()
makes datasets longer by increasing the number of rows and decreasing the number of columns.- Commonly needed to tidy wide datasets as they often optimise for ease of data entry or ease of comparison rather than ease of analysis.
-
pivot_wider()
makes a dataset wider by increasing the number of columns and decreasing the number of rows.- Relatively rare to need
pivot_wider()
to make tidy data, but it’s often useful for creating summary tables for presentation, or data in a format needed by other tools.
- Relatively rare to need
- For each of the sample tables, describe what each observation and each column represents.
table1
table1
table2
table2
table3
table3
- In each of
table1
,table2
, andtable3
, each observation represents a country. - In
table1
, country = country name, year = year of data collection, cases = number of people with the disease in that year, and population = number of people in each country in that year. - In
table2
, country and year are the same as intable1
, type = type of number, and count = number of observations (either cases or population depending on type). - Finally, in
table3
, country and year are again the same as intable1
, and rate = rate of disease (cases divided by population).
-
Sketch out the process you’d use to calculate the
rate
fortable2
andtable3
. You will need to perform four operations:-
Extract the number of TB cases per country per year.
-
Extract the matching population per country per year.
-
Divide cases by population, and multiply by 10000.
-
Store back in the appropriate place.
-
For table2
, we need to reshape the data to have a column for cases and a column for population and then divide the two to calculate the rate.
table2 |>
pivot_wider(
names_from = type,
values_from = count
) |>
mutate(rate = cases / population * 10000)
For table3
, we need to separate cases and population into their own columns and then divide them.
table3 |>
separate_wider_delim(
cols = rate,
delim = "/",
names = c("cases", "population"),
) |>
mutate(
cases = as.numeric(cases),
population = as.numeric(population),
rate = cases / population * 10000
)
Reference
Wickham, H., Mine Çetinkaya-Rundel and Grolemund, G. (2023) R for data science. 2nd ed. Sebastopol, CA: O’Reilly Media.